The explosion of AI has led to the creation of tools that make it more accessible, leading to more adoption and more numerous, less sophisticated users. As with cloud computing, that pattern of growth leads to misconfigurations and, ultimately, leaks. One vector for AI leakage is exposed Ollama APIs that allow access to running AI models.
Those exposed APIs create potential information security problems for the models’ owners. Of greater interest at the current moment, however, the metadata about the models, which also provides a gauge for the extent of DeepSeek adoption. By examining exposed Ollama APIs, we can see how AI users are already running DeepSeek in the U.S. and around the world.
Ollama background
Ollama is a framework that makes it easy to use and interact with AI models. Once a user installs Ollama, they are presented with a storefront of AI models. Ollama then installs and provides an API to interact with those models. In some cases, that API can be exposed to the public internet, making it possible for anyone to interact with those models.
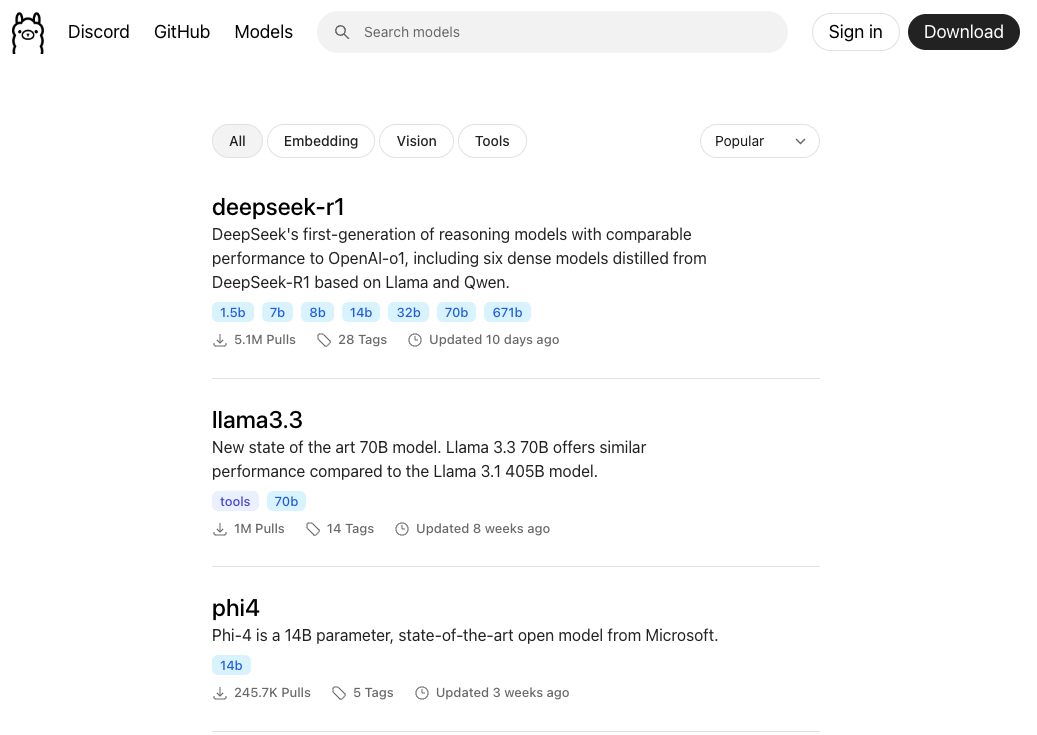
As others have noted before, this potential exposure is obviously quite risky. The Ollama API offers endpoints to push, pull, and delete models, putting any data in them at risk. Anonymous users can also make many generation requests to the models, running up the bill for the owner of the cloud computing account. Any vulnerabilities affecting Ollama may be exploited. Unauthenticated APIs exposed to the internet are bad.
Beyond the risks to the data security for the owners of these Ollama installations, exposing their model information provides us with visibility into the adoption of DeepSeek and its geographic distribution. The U.S. Navy, the state of Texas, NASA, and Italy have already banned the use of the DeepSeek app out of concerns that it leaks information to the Chinese government. In theory, those concerns would not apply to the open-source models where the code is available for inspection and modification by the person running it. However, users are unlikely to read the code, some researchers have found dangers in the model itself, and open-source projects can be backdoors for threat actors.
DeepSeek adoption
To measure the uptake of DeepSeek models in the U.S. and elsewhere, the UpGuard Research team analyzed the models running on exposed Ollama APIs, all of which reveal their running model names via unauthenticated APIs.
There are currently around seven thousand IPs with exposed Ollama APIs. In less than three months, that number has increased by over 70% since Laava performed a similar survey and found around 4,000 IP addresses with Ollama APIs.
Of the IPs currently exposing Ollama APIs, 700 are running some version of DeepSeek. 334 are running models in the deepseek-v2 family, which have been available for months. 434 are running deepseek-r1, released last week. Those numbers show that DeepSeek had already achieved significant adoption with their v2 family prior to the highly publicized R-1 model and that R-1 is growing far faster than any previous efforts from DeepSeek.
The highest concentration of IPs running one or more versions of DeepSeek is in China (171 IP addresses, or 24.4%). The second highest concentration is in the U.S., with 140 IP addresses, or 20%. Germany has 90 IP addresses, or 12.9%. The remaining IP addresses are distributed more or less evenly, with no other country having more than 5%.
.png)
The organizations owning those IPs are highly distributed. As mentioned, there are 140 IP addresses in the US are running DeepSeek on exposed Ollama APIs, and those are spread across 74 different organizations without strong concentration, even in cloud providers. These Ollama APIs are running on home or small business internet connections or university networks. These are most likely hobbyist deployments rather than corporate networks, which can be good and bad. If they are exploited, they would have a smaller blast radius, but they are also not subject to security programs (which is likely why they are configured this way in the first place). Home systems are less likely to be high-value targets in themselves but, conversely, are easier to compromise for use in botnets launching future attacks.
.png)
The deepseek-r1 models are more or less evenly distributed across the available parameter sizes, except for the most costly 671b parameter version. This distribution shows hobbyists' interest in fairly serious computing setups to support the 70 billion parameter version found in 75 IP addresses.
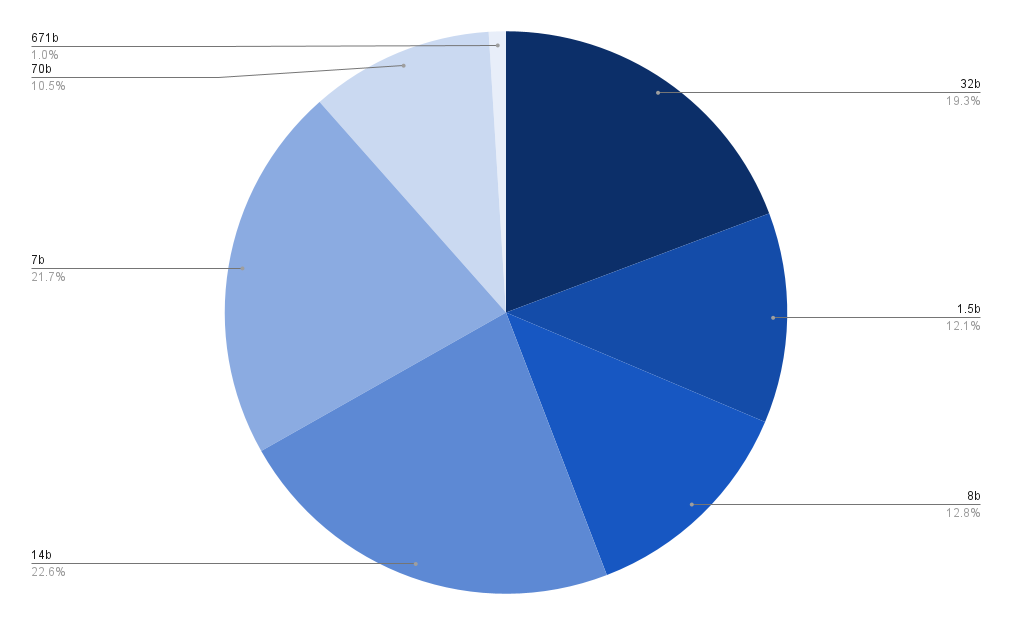
Indicators of compromise
In surveying exposed Ollama APIs, we observed some IPs with evidence of tampering. Whatever models were running have been replaced with one named “takeCareOfYourServer/ollama_is_being_exposed.” Based on past experience with similarly removed content from exposed databases and cloud storage buckets, this is most likely someone trying to help rather than a threat actor. (Ransom notes are not subtle and usually not so polite). Still, the theoretical risk of exposed models being downloaded, modified, or deleted is very real. These models and any data in them are at the mercy of the internet.
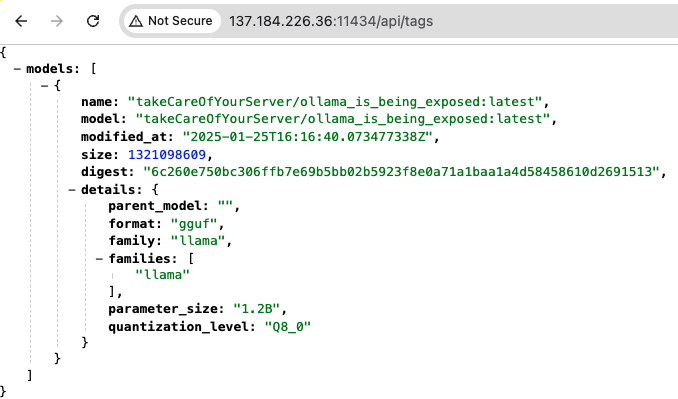
Conclusion
AI is everywhere, and individuals are adopting shadow AI even faster. UpGuard has more research coming out soon on this topic and our scanning engine already identifies which companies mention or use AI. Within days of the release of deepseek-r1, you could find it on misconfigured servers worldwide and almost certainly running in far more environments that we can’t observe through Ollama APIs.
Auditing one’s own attack surface and vendors for exposed Ollama APIs is the first step to prevent the most egregious form of AI data leakage. Beyond that, though, there are more questions to ask. As AI becomes a point of international competition, knowing which models or AI products your vendors use will need to be part of your third-party risk management program.